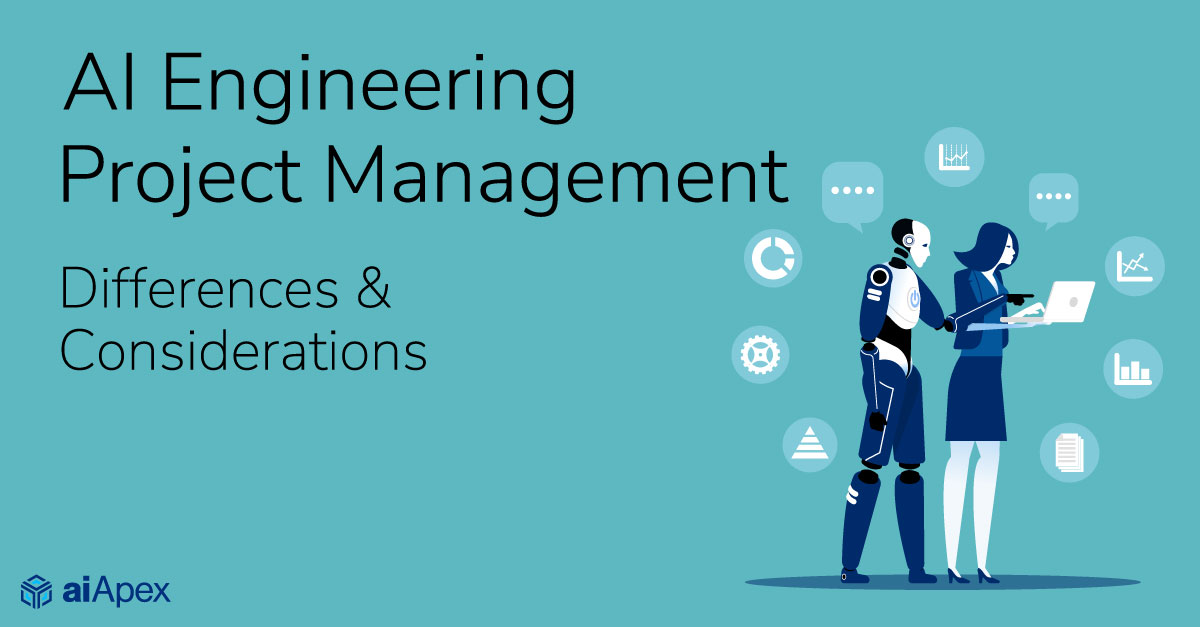
Managing AI Engineering Projects: Key Differences and Considerations
June 30, 2024
Introduction
Managing AI engineering projects presents unique challenges and considerations compared to standard enterprise transformation projects, such as implementing a CRM system. This article explores these differences, focusing on the AI project lifecycle, including data preparation, model development, and maintenance.
1. Project Initiation
AI Project:
- Problem Definition: Focus on defining the problem that the AI solution will address. This includes understanding the business objectives and identifying how AI can add value.
- Feasibility Study: Assess the feasibility of using AI to solve the problem, including data availability, quality, and the complexity of the AI models needed.
- Initial Use Case Identification: Identify initial use cases that will demonstrate the value of the AI solution, focusing on quick wins that can provide immediate business benefits.
Standard Enterprise Project (e.g., CRM Implementation):
- Project Charter: Define the project scope, objectives, stakeholders, and deliverables. This document sets the foundation for the project and aligns all stakeholders.
- Requirement Gathering: Collect business requirements from stakeholders to ensure the solution meets their needs.
- Feasibility Study: Assess the feasibility of implementing the solution within the existing infrastructure, focusing on technical and budgetary constraints.
2. Data Collection and Preparation
AI Project:
- Data Acquisition: Collect diverse and high-quality data from multiple sources, ensuring it is representative of the problem space.
- Exploratory Data Analysis (EDA): Conduct EDA to understand the data's structure, quality, and potential insights. This step is crucial for identifying patterns and determining the feasibility of using the data for machine learning models.
- Data Cleaning and Preparation: Clean and preprocess the data to make it suitable for model training. This includes handling missing values, outliers, and ensuring data consistency.
Standard Enterprise Project:
- Data Migration: Migrate data from legacy systems to the new system. This process often involves mapping data fields and ensuring data integrity.
- Data Cleaning: Clean and validate the data to ensure accuracy and consistency. This step is essential to avoid data-related issues in the new system.
- Data Mapping: Map data fields from old systems to new systems, ensuring that all necessary data is accurately transferred and available for use.
3. Development and Iteration
AI Project:
- Feature Engineering: Transform raw data into meaningful features for model training. This step is crucial for improving model performance.
- Model Training: Train machine learning models using the prepared data. This involves selecting appropriate algorithms and tuning hyperparameters.
- Iteration and Testing: Continuously test and refine models through iterative cycles to improve accuracy and performance. This process often requires multiple iterations to achieve the desired results.
- ML Ops: Implement ML Ops practices to manage the end-to-end machine learning lifecycle, including versioning, monitoring, and deployment. This ensures that models are consistently performing well in production.
Standard Enterprise Project:
- System Configuration: Configure the new system according to business requirements. This involves setting up system parameters and ensuring that the system behaves as expected.
- Customization: Develop custom features and functionalities as needed. This step may involve coding, scripting, or using development tools provided by the system vendor.
- Testing: Conduct unit, integration, and user acceptance testing to ensure the system meets requirements. This is essential to identify and fix any issues before deployment.
4. Validation and Evaluation
AI Project:
- Model Validation: Validate the performance of AI models using test datasets. This step ensures that the models generalize well to new, unseen data.
- Evaluation Metrics: Use specific evaluation metrics (e.g., accuracy, precision, recall) to assess model performance. Different metrics may be used depending on the problem type (classification, regression, etc.).
- Bias and Fairness Checks: Ensure the models are free from biases and make fair predictions. This is critical for maintaining ethical standards and regulatory compliance.
- Performance Monitoring: Implement monitoring to detect model drift and performance degradation over time. This involves setting up alerts and dashboards to track model performance metrics.
Standard Enterprise Project:
- System Validation: Validate the new system against business requirements and use cases. This step ensures that the system functions as intended and meets user needs.
- Performance Testing: Test the system’s performance under various conditions to ensure it can handle expected workloads.
- User Training: Train end-users on the new system to ensure smooth adoption. This often involves creating training materials and conducting training sessions.
5. Deployment and Integration
AI Project:
- Model Deployment: Deploy the trained models into production environments. This involves setting up inference pipelines and ensuring that models can make real-time predictions.
- Integration with Systems: Integrate AI models with existing enterprise systems and workflows. This ensures that the AI solutions are seamlessly incorporated into business processes.
- Continuous Monitoring: Continuously monitor model performance and make necessary adjustments to maintain accuracy. This involves regularly retraining models with new data and addressing any issues that arise.
Standard Enterprise Project:
- System Deployment: Deploy the new system into production. This involves setting up the system in the live environment and ensuring it is ready for use.
- System Integration: Integrate the new system with other enterprise applications. This ensures that data flows smoothly between systems and that business processes are not disrupted.
- Go-Live Support: Provide support during the initial go-live phase to resolve any issues. This is essential to ensure a smooth transition to the new system.
6. Maintenance and Evolution
AI Project:
- Model Maintenance: Regularly update and retrain models with new data to maintain performance. This ensures that models remain accurate and relevant as business conditions change.
- Continuous Improvement: Continuously improve models based on feedback and changing business conditions. This involves experimenting with new algorithms and techniques to enhance model performance.
- Adaptability: Ensure the AI solution can adapt to new data sources, changing business requirements, and evolving technologies. This involves designing flexible and scalable solutions.
Standard Enterprise Project:
- System Maintenance: Provide regular updates and maintenance to ensure the system operates smoothly. This involves applying patches, updates, and addressing any technical issues.
- Feature Enhancements: Develop and deploy new features and enhancements based on user feedback and evolving business needs. This ensures that the system continues to meet user requirements.
- Support and Troubleshooting: Offer ongoing support to address any issues or challenges users may face. This ensures that users can effectively use the system and that any problems are quickly resolved.
7. Challenges and Solutions
Data Management
AI projects present unique challenges that require tailored solutions:
- Data Privacy and Security: Ensuring data privacy and security is paramount in AI projects. This involves implementing robust security measures and complying with data protection regulations.
- Integration with Existing Systems and Human Workforce: Integrating AI solutions with existing enterprise systems and workflows can be complex. This requires careful planning and coordination to ensure seamless integration.
Resource Management
AI projects require specialized skills and expertise:
- Specialized Skills and Expertise: AI engineering requires specialized skills in data science, machine learning, and AI development. This may involve hiring or training personnel with the necessary expertise.
- Team Collaboration: Cross-functional collaboration between data scientists, engineers, domain experts, and business stakeholders is critical for project success. This ensures that all perspectives are considered and that the solution meets business needs.
Conclusion
AI engineering projects differ significantly from standard enterprise projects, requiring a strategic and methodical approach. Understanding these unique challenges and implementing best practices in data management, model training, and ML Ops are key to successfully managing AI projects and achieving desired outcomes. By focusing on the distinct lifecycle stages and addressing the specific challenges of AI development, organizations can effectively leverage AI to drive business value and innovation.
If you would like to discuss our perspectives, our responses to these challenges for your organization, and our approach to Enterprise AI, please contact us using the form below or at [email protected].